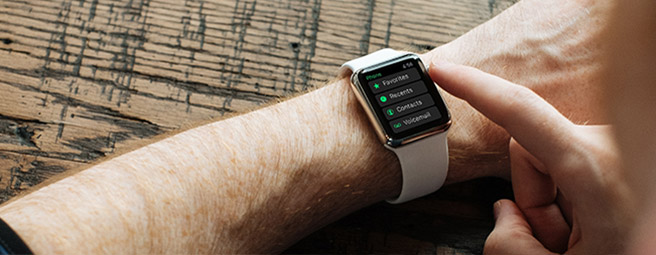
Using Natural Language Processing for Deeper and Broader Customer Support Insights
There are a lot of customer service channels available today. Customers expect multichannel experiences and the ability to engage via their channel of preference. Businesses that fail to offer their customers omnichannel support options are at competitive disadvantages. Some of the ways that customers expect businesses to engage with them when it comes to support include:
• Phone
• Live Web Chat
• Social Media
• Mobile Apps
• Chatbots
• Online Knowledgebase
• Online Communities—Company and Third Party
• Support Portals
Customers also want to use any number of devices, with mobile—smartphones and now smartwatches—transforming when and where engagement takes place.
Intelligent Chatbots
The implications of not offering customers the support engagement channels they want to use isn’t only confined to enterprises. Technology disruption has shrunk the barriers to entry so that small businesses can tap into multichannel support delivery just as quickly and easily as much larger enterprise counterparts. And businesses don’t even need to have staff in place to add these support channels to their portfolio with the availability of managed services such as Davinci’s Live Receptionist and Live Web Chat services and automated chatbot-like services like Davinci Auto Receptionists.
Chatbot support engagement is the hottest topic and trend today. The accuracy of natural language processing (NLP) and machine learning—namely, the ability to understand human language—has improved dramatically (with a word error rate of less than 5% for many). As these chatbots improve in identifying behavioral intent, the capabilities of these support chatbots will improve further, providing customers with human-like support conversations.
Possibilities of NLP
NLP offers organizations a broad range of support opportunities. Chatbots are just one area. When each of the different customer engagement channels are considered, the breadth and depth of structured and unstructured data that businesses capture is substantial. However, according research firm IDC, upwards of 80 percent of customer data goes unanalyzed. There are certainly numerous use-case scenarios—both within support organizations and across organizations—when it comes to business insights that can be derived from the analysis of customer data:
• Customer sentiment in aggregate and across each support engagement channel
• Top issues ranked in terms of sentiment polarity and/or frequency of occurrence
• Persona development based on needs and values analysis, personality types, language, social, and emotion tone analyses
Individually or in total, these can be used to deliver better service, faster identification and remediation of product or support issues, more effective marketing experiences, and develop products that resonate with customers better.
Customer data is defined in terms of three factors: volume (amount), velocity (rate with which information changes), and variety (range and types of data). As each of these increase, the depth and breadth of insights expand in scope, revealing hidden patterns and relationships. Analytics can be organic whereby the data determines the actual areas of business insight, or forensic whereby a specific topic or issue is examined.
When it comes to the actual analysis of data, there are four different models:
1. Descriptive. Looks at data based on what is happening now and develops business insights that reflect the status quo.
2. Predictive. Looks at data through the lens of future scenarios (what might happen) and provides a predictive model that forecast these potential use-case outcomes.
3. Diagnostic. Looks at past performance to determine what happened and why.
4. Prescriptive. Deeper analysis that overlays different data sources for cause-effect scenarios that are used to formulate specific rules and recommendations.
NLP in Action
Key-phrase (or topic) analysis augmented with sentiment polarity is a common form of NLP. Using Cognition Insights, a technology-enabled managed service, TIRO Communications has worked with clients across multiple industry segments to pinpoint actionable business insights. Let’s look at some of the recommendations we’ve given clients based on key-phrase and sentiment analysis as well as advanced persona development.
Example One. A software client discovered that its sentiment polarity is much higher than that reflected on external review sites in comparison to competitors (viz., rating scales of 1 – 5 or 1 – 10 are subjective whereas NLP is based on customer language). The same client also honed its customer advocacy program, identifying topics where additional reviews are needed and opportunities where reviews provide competitive advantage.
Example Two. Another client in the unified communications sector thought it had a problem with audio quality. The company did not know to what extent, and how it stacked up against key competitors. Cognition Insights helped them understand the frequency and types of audio quality mentions by customers and competitor customers (forensics analysis). It also revealed the nature of support issues, which the client prioritized based on the extent of the problem and ease of resolution.
Example Three. A software client wanted to base its demand-gen campaign calendar for the year on the top topics identified by key-phrase and sentiment analysis. Competitive comparison revealed topics where the client had the greatest likelihood to displace individual competitors.
Example Four. A client offering consultative services to global enterprises wanted to improve the effectiveness of its demand-gen and sales initiatives. Analyzing the natural language of buyers for its top 40 accounts, the client derived and assigned a persona grid to each buyer (a matrix of 192 possibilities) to fine tune language tone in sales outreach emails based on those assignments and to identify content types most likely to resonant with each buyer.
One of the most obvious takeaways from the above examples is the fact that customer support data provides actionable insights across multiple organizational functions. Another takeaway is that the same data can yield different types of insights for different business areas. For example, key-phrase and sentiment analysis can uncover business process support issues, product quality areas for remediation, and competitive strengths, weaknesses, opportunities, and threats (SWOT) for sales battle-card development.
NLP is creating some exciting opportunities for customer support. Organizations large and small are leveraging the data gathered across each engagement channel to create business insights that deliver tangible value that includes identification and remediation of support and product problems, more effective marketing campaigns, better content utilization rates, segmented customer advocacy activities, and communications and content that align with customer personas.
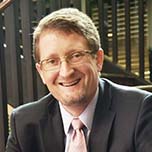
LinkedIn Profile: LinkedIn
Website: www.tirocommunications.com
Categories
- Business Marketing
- Business Resources
- Customer Success Stories
- Customer Success Stories: Communications
- Customer Success Stories: Meeting Rooms
- Customer Success Stories: Virtual Office
- Entrepreneur Tools
- Management
- Meeting Rooms
- Virtual News
- Virtual Office
- Virtual Receptionist Services
- Workplace Culture
Subscribe to Our Blog
Archive
- July 2024
- June 2024
- May 2024
- April 2024
- March 2024
- February 2024
- January 2024
- December 2023
- November 2023
- October 2023
- September 2023
- August 2023
- July 2023
- June 2023
- May 2023
- April 2023
- March 2023
- February 2023
- January 2023
- December 2022
- November 2022
- October 2022
- September 2022
- August 2022
- July 2022
- June 2022
- May 2022
- April 2022
- March 2022
- February 2022
- January 2022
- December 2021
- November 2021
- October 2021
- September 2021
- August 2021
- July 2021
- June 2021
- May 2021
- April 2021
- March 2021
- February 2021
- January 2021
- December 2020
- November 2020
- October 2020
- September 2020
- August 2020
- July 2020
- June 2020
- May 2020
- April 2020
- March 2020
- February 2020
- January 2020
- December 2019
- November 2019
- October 2019
- September 2019
- August 2019
- July 2019
- June 2019
- May 2019
- April 2019
- March 2019
- February 2019
- January 2019
- December 2018
- November 2018
- October 2018
- September 2018
- August 2018
- July 2018
- June 2018
- May 2018
- April 2018
- March 2018
- February 2018
- January 2018
- December 2017
- November 2017
- October 2017
- September 2017
- August 2017
- July 2017
- June 2017
- May 2017
- April 2017
- March 2017
- February 2017
- December 2016
- November 2016
- October 2016
- September 2016
- August 2016
- July 2016
- June 2016
- May 2016
- April 2016
- March 2016
- February 2016
- January 2016
- December 2015
- November 2015
- September 2015
- August 2015
- July 2015
- June 2015
- April 2015
- March 2015
- February 2015
- January 2015
- December 2014
- November 2014
- October 2014
- September 2014
- August 2014
- July 2014
- May 2014
- April 2014
- March 2014
- January 2014
- December 2013
- October 2013
- September 2013
- August 2013
- June 2013
- April 2013
- February 2013
- January 2013
- December 2012
- November 2012
- October 2012
- September 2012
- August 2012
- July 2012
- June 2012
- May 2012
- April 2012
- March 2012
- February 2012
- January 2012
- December 2011
- November 2011
- October 2011
- September 2011
- April 2011
- March 2011
- January 2011
- December 2010
- October 2010
- September 2010
- July 2010
- June 2010
- April 2010
- March 2010
- January 2010
Talk to an expert
Want to know more about our Virtual Offices? Give us a call
Get the Perfect Virtual Address Now
Find a Virtual OfficeServices
Virtual Offices Virtual Office Features What is a Virtual Office? Full Time Spaces Live Receptionist Live Receptionist Features Auto Receptionist Live Web Chat Meeting Spaces Meeting Space Features Coworking Spaces Event Spaces Marketplace Incorporation Services Search Virtual Office Services Near MeInstant Group
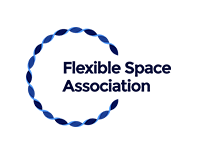
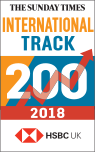
- © 2024 Davinci virtual office